This AI paper studies test time scaling of English-centric RLM to enhance multilingual reasoning and domain summary

Inference language models, or RLM, are increasingly used to gradually solve problems by generating long structured inference chains. These models break down complex problems into simpler parts and build logical steps to achieve the answer. This operational chain (COT) approach has proven to be effective in improving output quality, especially in mathematical and logical tasks. Despite the multilingual functions in many modern large models, the focus of research and training remains largely focused on English, leaving gaps in understanding how these inference skills translate into other languages.
A major challenge is that most RLMs are fine-tuned on English data, which limits their ability to reason effectively in other languages. This is especially problematic for low-resource languages with limited training examples. These models may default to English thinking mode, producing lower quality output when prompted in another language. Furthermore, differences in language structures can lead to inference errors, especially when a model trained in one language will infer logic in another language without sufficient linguistic consistency.
Current technology uses zero beats or several-motivation strategies to manage these limitations, often in English as the pivot language. Some efforts involve preserving language consistency with cues in the same language as query. However, due to limited capacity, small models have minimal benefits, and even large models can show inconsistent performance when inferring low-resource languages. Despite multiple language pre-reading, the gap between training languages and inference languages continues to hinder accurate multilingual reasoning.
Brown University and the MBZUAI research team are committed to assessing the increase in increased test time calculations, especially through how extended chains of reasoning affect multilingual reasoning capabilities of English-centric RLMs. They conducted a survey using the S1 model based on the QWEN2.5 teaching structure and fine-tuned 1,000 samples of English STEM reasoning. These models were tested using benchmarks such as MGSM and Global-MMLU to answer four core questions: cross-language testing time scaling, language mixing behavior, performance under language effectiveness, and effectiveness of cross-domain generalization.
In-depth experiments show that models with more parameters can significantly benefit from testing time thinking tokens. When the 14B S1 model scales to 8,000 mind tokens, the average accuracy of non-English languages is 81% in MGSM. It outperforms the +23.1% French teaching in QWEN2.5-14B, while the model in Swahili exceeds +23.1%. Even though the model is trained in English only, its performance outperforms larger models, such as DeepSeek’s R1-Distill-Qwen-32b using several high-resource languages. The study also found that inferences in high-resource languages such as Chinese and English are more effective in low-resource languages such as Swahili or Telugu, requiring fewer tokens and better results.
A key observation is the behavior of “citation and thinking”, which cited non-English phrases in the prompt and reasoned in English. This consistent pattern across languages such as Japanese and Russian suggests that the model uses its multilingual understanding to interpret non-English input without direct translation. Experiments on language stimulation further confirmed that inferences that forced high-resource languages yielded better results, while strict inferences of low-resource languages resulted in significant reductions in accuracy and inefficiency in computational efficiency.
Despite strong results in STEM-related tasks, performance improvements did not shift to areas such as cultural common sense or humanities. In benchmarks such as forks, adding a mind token sometimes degrades performance, indicating overthinking. The study concluded that although test time scaling enhances multilingual reasoning for high-source languages, it was not effectively generalized to outdoor tasks or low-resource languages, suggesting the need for further research on balanced multilingual training and domain adaptation.
Check Paper. All credits for this study are to the researchers on the project. Also, please feel free to follow us twitter And don’t forget to join us 90K+ ml reddit.
Here is a brief overview of what we built in Marktechpost:
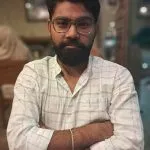
Nikhil is an intern consultant at Marktechpost. He is studying for a comprehensive material degree in integrated materials at the Haragpur Indian Technical College. Nikhil is an AI/ML enthusiast and has been studying applications in fields such as biomaterials and biomedical sciences. He has a strong background in materials science, and he is exploring new advancements and creating opportunities for contribution.