This AI paper introduces MMADA: a unified multimodal diffusion model for text reasoning, visual understanding, and image generation

The diffusion model known for successfully generating high-quality images is now being explored as the basis for handling multiple data types. These models Denoise data and reconstruct the original content from noisy input. This capability makes diffusion models promising for multimodal tasks involving discrete data, such as text and continuous data, such as images.
The challenge of multimodal models is to build systems that can handle the understanding and generation of text and images without using separate methods or architectures. Existing models often struggle to balance these tasks effectively. They are designed for specific tasks, such as image generation or question answering, which leads to limited performance for unified tasks. The post-training technology that can be further adjusted in inference and power generation tasks is also underdeveloped, leaving a gap in fully integrated multi-models that can be used to meet various challenges using a single design.
Popular methods such as Show-O, Janus, and Seed-X combine autoregressive models for text and diffusion models for images, requiring separate loss functions and architectures. These models use different tokenization schemes and separate pipelines for text and image tasks, complicating training and limiting their ability to handle reasoning and generation in a unified way. Furthermore, they focus on training strategies, ignoring post-training methods that can help these models learn to reason on different data types.
Researchers at Princeton University, Peking University, Tsinghua University and Bytedance have launched MMADA, a unified multi-modal diffusion model. The system integrates text reasoning, visual understanding, and image generation into a probability framework. MMADA simplifies training across different data types using shared diffusion architectures without relying on schema-specific components. The model is designed to process text and visual data together, thus providing a simplified, cohesive approach to inference and generation tasks.
The MMADA system introduces a hybrid long-term, thought-out (long-term) fixed strategy that aligns the inference steps across text and image tasks. Researchers curated a diverse set of data sets of inference traces, such as problem-solving of mathematical and visual problems, to guide models to learn complex inferences across modalities. They also developed Unigrpo, an enhanced learning algorithm tailored to diffusion models that uses policy gradients and diversified reward signals, including correctness, format compliance, and consistency with visual content. The model’s training pipeline combines a unified masking strategy with structured deoing steps to ensure stability during the learning process and allows the model to effectively reconstruct content across different tasks.
In performance benchmarks, MMADA has shown good results in various tasks. For text-to-image generation, it has a clip score of 32.46, and the imaging-to-1.15 clip score is better than models such as SDXL and Janus. In the multimodal understanding, it reaches a Pope score of 86.1, with an MME score of 1410.7, while the FlickR30k scores of 67.6, surpassing systems like Show-O and Seed-O and Seed-X. For text reasoning, MMADA scored 73.4 on GSM8K and 36.0 on Math500, outperforming other diffusion-based models such as LLADA-8B. These results highlight MMADA’s ability to provide consistent, high-quality output in reasoning, understanding and power generation tasks.
Overall, MMADA solves the challenge of building a unified multi-model by introducing simplified architecture and innovative training techniques. Research shows that diffusion models can be excellent as a general-purpose system capable of inference and generation across multiple data types. By addressing the limitations of existing models, MMADA provides a blueprint for the development of future AI systems that seamlessly integrate different tasks into a powerful framework.
View paper, model on hug surface and github page. All credits for this study are to the researchers on the project. Also, please stay tuned for us twitter And don’t forget to join us 95k+ ml reddit And subscribe Our newsletter.
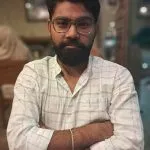
Nikhil is an intern consultant at Marktechpost. He is studying for a comprehensive material degree in integrated materials at the Haragpur Indian Technical College. Nikhil is an AI/ML enthusiast and has been studying applications in fields such as biomaterials and biomedical sciences. He has a strong background in materials science, and he is exploring new advancements and creating opportunities for contribution.
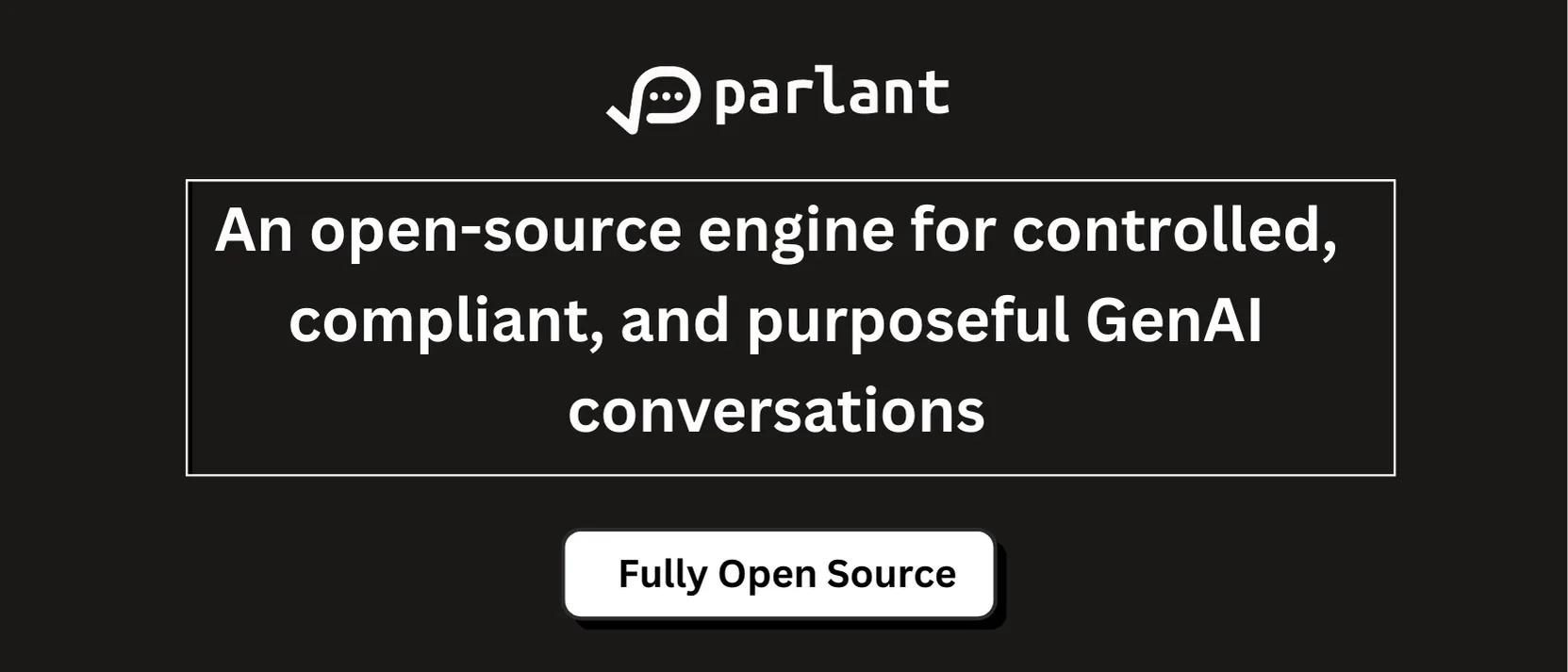