Salesforce AI researchers introduce UAEVAL4RAG: A new benchmark for evaluating queries that are rejected by rag systems that cannot be answered

While RAGs can respond without extensive model retraining, the current evaluation framework will focus on the accuracy and relevance of answerable questions, ignoring the critical ability to reject inappropriate or unanswerable requests. This creates high risk in real-life applications where inappropriate responses can lead to misinformation or harm. Existing unselectable benchmarks are insufficient for rag systems because they contain static, general requests that cannot be customized for a specific knowledge base. When a rag system rejects a query, it usually stems from a search failure rather than a real recognition that some requests should not satisfy some, thereby highlighting the key gap in the evaluation method.
Unanswered benchmark studies provide insights about model non-compliance, exploring ambiguous questions, and specifying inputs. RAG evaluation has advanced through different LLM-based technologies, with methods such as Ragas and Ares evaluating the relevance of retrieved documents, while RGB and Multihop-rag focus on output accuracy for ground truth. In unanswered rag evaluation, some benchmarks have begun to evaluate repulsion in rag systems, but use the unanswered background generated by the LLM as external knowledge and narrowly evaluate the repulsion of single-type untouchable requests. However, current methods do not adequately evaluate the ability of the rag system to reject various unanswered requests between user-provided knowledge bases.
Researchers at Salesforce Research proposed UAEVAL4RAG, a framework designed to synthesize unanswered requested datasets about any external knowledge database and to automatically evaluate the rag system. The UAEVAL4RAG not only evaluates that the rag system responds well to answerable requests, it also rejects six different categories of unanswered queries: unspecified, false manifestations, non-sensitivity, meaninglessness, modal limitations, security issues, security issues and scope. The researchers also created an automated pipeline that generates diverse and challenging requests designed for any given knowledge base. The generated data set is then used to evaluate a rag system with two LLM-based metrics: unanswered ratios and acceptable ratios.
UAEVAL4RAG evaluates how different rag components affect the performance of responsive and unanswerable queries. After testing a combination of 27 embedding models, search models, rewrite methods, rewriters, 3 LLMs and 3 prompt techniques, the results show that due to different knowledge distributions, there is no single configuration optimized for all datasets. The choice of LLM proved crucial, with Claude 3.5 sonnets improving accuracy by 0.4%, while the unapproachable acceptable ratio increased by 10.4% over the GPT-4O ratio. Timely design will affect performance, and the best tips will increase the performance of unanswered queries by 80%. In addition, three metrics evaluate the ability of the rag system to reject unanswered requests: acceptable ratios, unresolved ratios, and joint scores.
UAEVAL4RAG is highly effective in generating unanswered requests, with the Triviaqa and Musique datasets having an accuracy of 92% and a strong ratings consistency score of 0.85 and 0.88. The LLM-based metrics demonstrate good performance and have high accuracy and F1 scores on three LLMSs, verifying their reliability in evaluating rag systems regardless of the backbone model used. Comprehensive analysis shows that a single combination of rag components is good at all datasets, and timely design can affect hallucination control and query rejection capabilities. Dataset characteristics with schema-dependent performance are correlated with keyword prevalence (18.41% for Triviaqa and 6.36% for HOTPOTQA), and request processing for security based on block availability for each issue.
In summary, the researchers introduced UAEVAL4RAG, a framework for evaluating the ability of rag systems to handle unanswered requests, addressing the key gaps in existing evaluation methods that focus primarily on answerable queries. Future work may increase generalizability for more diverse sources of human verification. Although the proposed indicators show strong consistency with human assessments, customizing them to a specific application can further improve effectiveness. Current evaluation focuses on single-turn interactions, while extending the framework to multi-turn dialogue will better capture real-world scenarios where the system participates in communicating with users to manage unspecified or ambiguous queries.
View paper. All credits for this study are to the researchers on the project. Also, please feel free to follow us twitter And don’t forget to join us 95k+ ml reddit And subscribe Our newsletter.
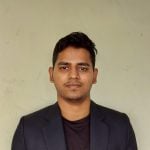
Sajjad Ansari is a final year undergraduate student from IIT Kharagpur. As a technology enthusiast, he delves into the practical application of AI, focusing on understanding AI technology and its real-world impact. He aims to express complex AI concepts in a clear and easy way.
🚨Build a Genai you can trust. ⭐️Parlant is your open source engine for controlled, compliance and purposeful AI conversations – Star Parlant on Github! (Promotion)