Meta AI introduces multi-space Mllm: multi-frame space understanding with multi-modal large language model

Multimodal Large Language Model (MLLM) shows great progress because of the multi-functional AI assistant that can handle a variety of visual tasks. However, their deployment as siloed digital entities limits their potential impact. The growing demand for integrating MLLM into real-world applications such as robotics and autonomous vehicles requires complex spatial understanding. Current MLLMs show basic spatial reasoning flaws, which usually fail on basic tasks such as distinguishing left and right. Although previous studies attribute these limitations to insufficient professional training data and address these limitations through spatial data during training, these approaches focus on single-image scenarios, thus limiting the model’s perception of static field analysis without dynamic information.
Several research methods attempt to address spatial understanding limitations in MLLM. MLLMS incorporates image encoders that convert visual input into tokens processed with text in the latent space of the language model. Previous research focused on single-image spatial understanding, evaluating spatial relationships or spatial recognition among objects. Some benchmarks, such as blink, uniqa-3D, and VSibench, go beyond a single image. Existing improvements to MLLM for spatial understanding, including spatial VLM, which fine-tunes the model on curated spatial datasets, “spatial” that combines mask-based reference and depth images and spatially Spatialpin, which utilizes a dedicated perceptual model without fine-tuning.
Researchers from Fair Meta and the University of China in Hong Kong have proposed a framework to enhance MLLM to gain a strong multi-frame space understanding. This integrates three components: depth perception, visual correspondence and dynamic perception to overcome the limitations of static single image analysis. The researchers developed MultiSPA, a new large dataset containing more than 27 million samples covering different 3D and 4D scenarios. The resulting multi-space model model provides significant improvements to benchmarks and proprietary systems with scalable and generalizable multi-frame inference. In addition, five tasks were introduced to generate training data: depth perception, visual correspondence, camera motion perception, object motion perception, and object size perception.
Multi-space passwords revolve around multi-motion data generation pipelines and a comprehensive benchmark system. The data format follows a standard MLLM fine-tuning strategy, which has a QA pair format: User:
In the MultiSPA benchmark, the base model of multispace Mllm grew by an average of 36%, achieving 80-90% accuracy on qualitative tasks, while the baseline model exceeded 50%, while performing better than all proprietary systems. Even in challenging tasks such as predicting camera motion vectors, it achieves 18% accuracy with near-zero performance on other baselines. On the blink benchmark, multi-space molme accuracy is close to 90%, an average of 26.4% improvement over the basic model, surpassing several proprietary systems and showing a passable multi-frame space understanding. Standard VQA benchmark evaluation shows that parity of the original performance is rough, indicating that the model maintains the ability of general-purpose MLLM and is not suitable for spatial reasoning tasks.
In this article, the researchers extend the spatial understanding of MLLMS to multi-frame scenarios, addressing key gaps overlooked in previous studies. They introduce MultiSPA, the first large-scale dataset and benchmark for multi-framework spatial inference tasks. Experimental verification shows the effectiveness, scalability and powerful generalization capabilities of multispace millimeters proposed in various spatial understanding challenges. The study reveals important insights, including the benefits of multitasking learning and emerging behaviors in complex spatial reasoning. The model builds new applications, including acting as a multi-frame reward commenter.
View paper, project pages, and GitHub pages. All credits for this study are to the researchers on the project. Also, please stay tuned for us twitter And don’t forget to join us 95k+ ml reddit And subscribe Our newsletter.
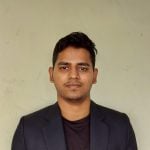
Sajjad Ansari is a final year undergraduate student from IIT Kharagpur. As a technology enthusiast, he delves into the practical application of AI, focusing on understanding AI technology and its real-world impact. He aims to express complex AI concepts in a clear and easy way.
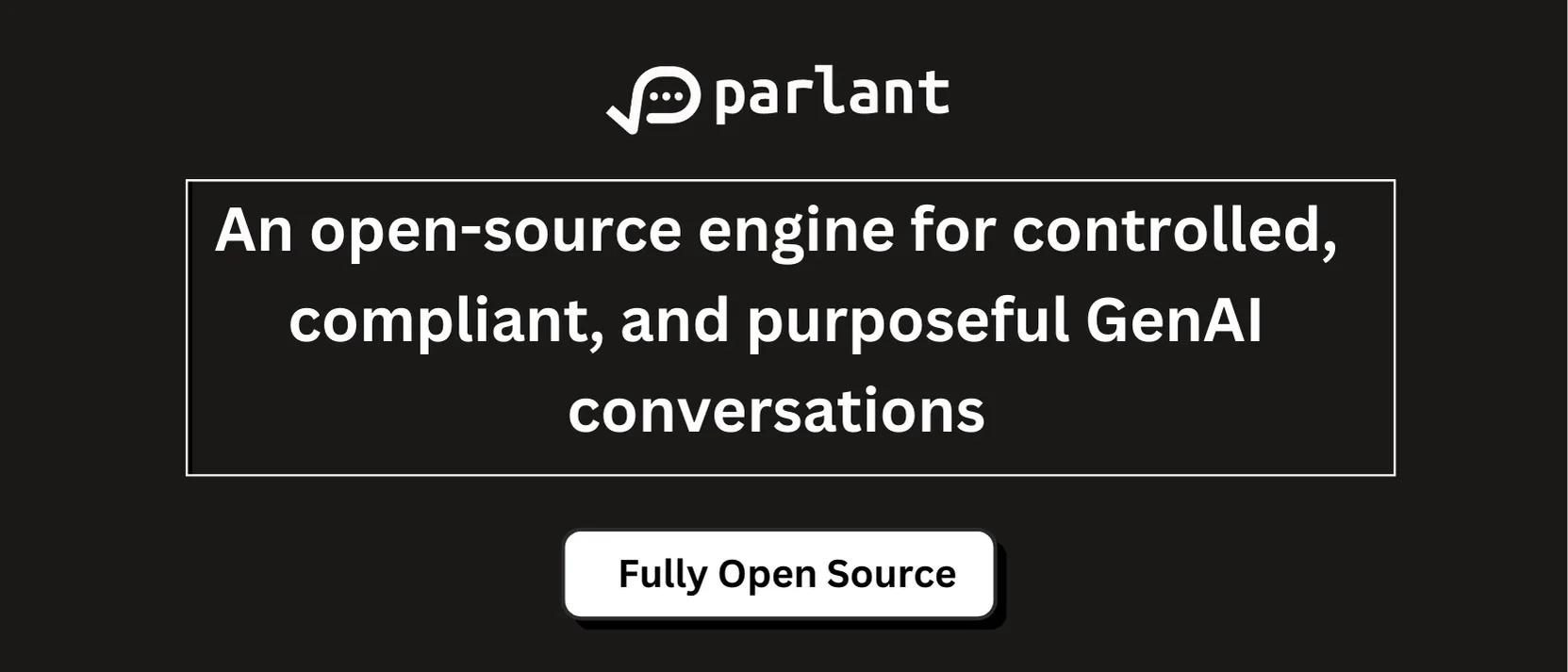