DanceGrpo: A unified framework for visually generated enhanced learning across multiple paradigms and tasks

Generative models, especially diffusion models and rectifier flows, revolutionize the creation of visual content with enhanced output quality and versatility. Human feedback integration during training is critical to align output with human preferences and aesthetic standards. Current methods, etc., depend on distinguishable reward models, which introduces inefficient VRAM generated by videos. DPO variants only achieve marginal visual improvements. Furthermore, RL-based approaches face challenges, including conflicts between ODE-based process model sampling and Markov decision-making process formulas, instability when scaling beyond small datasets, and lack of validation of video generation tasks.
Aliging LLMS employs reinforcement learning from human feedback (RLHF), which trains reward functionality based on comparative data to capture human preferences. The policy gradient approach has been proven to be effective but is effective in terms of computational intensiveness and requires extensive adjustments, while Direct Policy Optimization (DPO) provides cost-efficient but low performance. DeepSeek-R1 recently showed that large-scale RL with special rewards features can guide LLMS’s thought process towards self-emission. Current methods include DPO-style approaches, direct backpropagation of reward signals such as reward signals, and policy gradient-based methods such as DPOK and DDPO. Due to the unstable policy gradient approach in large-scale applications, production models mainly utilize DPO and REFL.
Researchers from Bondedance Seed and the University of Hong Kong proposed DanceGrpo, a unified framework that adapts to the group’s relative policy optimization to the optimization of visual generation paradigm. The solution runs seamlessly in diffusion models and rectifying streams, processing text-to-image, text-to-video and image-to-video tasks. The framework is integrated with four basic models (Stable Diffusion, Hunyuanvideo, Flux, Skyreels-I2V), and five reward models cover image/video aesthetics, text image alignment, video motion quality and binary reward evaluation. DanceGrpo has a benchmark of up to 181% in key benchmarks including HPS-V2.1, Clip Score, VideoAlign and Geneval.
The architecture utilizes five professional reward models to optimize visual generation quality:
- Image Aesthetics Use models that fine-tune the human hierarchy data to quantify visual appeal.
- Text image alignment Use clips to maximize cross-mode consistency.
- Video aesthetic quality Use the Visual Language Model (VLM) to extend the evaluation to the time domain.
- Video motion quality Evaluation of motion realism through physical consciousness VLM analysis.
- Threshold binary reward A discrete mechanism is adopted where values exceeding the threshold receive 1 and other 0, specifically designed to evaluate the ability of generative models to learn sudden reward distributions under threshold-based optimization.
DanceGrpo showed a significant improvement in the reward metric for steady diffusion v1.4, with HPS scores improved from 0.239 to 0.365, while clip scores increased from 0.363 to 0.395. Pick-A-PIC and Geneval evaluations confirmed the effectiveness of the method, while DanceGrpo performed better than all competing methods. For Hunyuanvideo-T2i, optimization using the HPS-V2.1 model increased the average reward score from 0.23 to 0.33, showing enhanced consistency with human aesthetic preferences. For HunyuanVideo, although text video alignment was excluded due to instability, the relative improvements of this method achieved 56% and 181% improvements in visual and motion quality metrics, respectively. DanceGrpo uses the motion quality metrics of the video reward model, achieving a relative improvement of 91% in this dimension.
In this article, researchers have introduced DanceGrpo, a unified framework for enhancing the cross-text model and rectified streams across text to images, text to videos, and image to videos. It addresses the critical limitations of previous approaches by bridging the gap between language and visual modes, thus achieving superior performance through effective maintenance and robust multitasking setups with human preferences. Experiments show that visual fidelity, motion quality, and text image alignment have greatly improved. Future work will explore the expansion of GRPO to multi-modal generation, further unifying the optimization paradigm for generating AI.
Check Paper and project page. All credits for this study are to the researchers on the project. Also, please feel free to follow us twitter And don’t forget to join us 90K+ ml reddit.
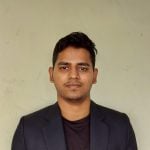
Sajjad Ansari is a final year undergraduate student from IIT Kharagpur. As a technology enthusiast, he delves into the practical application of AI, focusing on understanding AI technology and its real-world impact. He aims to express complex AI concepts in a clear and easy way.
