The latest advances in long-form culture (LC) modeling have unlocked new features for LLM and large-scale visual models (LVLMS). Long context vision – Language Model (LCVLM) takes an important step forward by enabling LVLMS to process hundreds of images and thousands of interwoven text tokens in a single forward pass. However, the development of effective evaluation benchmarks lags. It is unclear how the current LCVLM performs in novel settings, how robust the tasks they encounter, and how change in input lengths are. Current benchmarks face the following problems: (a) limited coverage for downstream tasks, (b) insufficient coverage for image types, (c) lack of context length control, and (d) single context length.
Various technologies have context windows that extend LVLM, including longer pretrained lengths, position extrapolation and efficient architecture. Models such as Gemini-2.5 and QWEN2.5-VL have adopted these methods, as well as visual token compression methods, to accommodate longer sequences. For evaluation, the needle-wire-range task in the needle has become a standard benchmark for testing LC capabilities by inserting information in a specific depth within long text. However, existing visual benchmarks are still limited, focusing only on NIAH variants or long-term document VQA tasks. Even Milebench contains short context tasks with an average length of just 9K tokens, and cannot evaluate true LC capabilities in various visual language applications.
Researchers from HKUST, Tencent AI Seattle Laboratory, University of Edinburgh, Miniml.ai and Nvidia AI Technology Center have proposed Mmmlongbench, the first comprehensive benchmark for evaluating LCVLMS. It includes 13,331 examples covering five downstream task categories, including visual rags and many shooting ICLs, covering both natural and synthetic image types. All examples are normalized using a cross-mode token scheme combining visual plaques and five input lengths of text tokens. By benchmarking 46 closed and open source models, the study shows that single-task performance predicts overall LC functionality, both model types struggle with LC tasks, and a more powerful inference model shows better LC performance.
The researchers constructed the LC by inserting golden segments containing answers into a large number of distraction paragraphs retrieved by Wikipedia. For Viquae, the golden channel of the kilt is used, while Infoseek uses the lead portion of the Wikipedia entity page. Additionally, the Wikipedia page is divided into 100-word paragraphs and the retrieved jammer is added until the desired input length is reached. Many internal learning tasks utilize four different image classification datasets: Stanford, Food101, Sun397, and Inat2021, which can accommodate 500 images in a 128K context window. Cross-mode token counting combines text tokens using Llama2 tokens and unredeemable compressed visual tokens through 14×14 patches and 2×2 pixels, ensuring compatibility with modern LVLMS.
Evaluation of Mmlongbench across tasks and context lengths shows that all models are working hard, but closed source models perform better. For the longest 128K input length, all models involved long cultural visual tasks, with the GPT-4O reaching only 62.9 average performance. Gemini-2.5-Pro became the strongest performance, with an open source model of more than 20 points in addition to ICL tasks. Furthermore, the OVIS2-34B model scored 41.6 in the summary, similar to GPT-4O (42.4). QWEN2.5-VL-32B has a SUBEM score of 64.6 on VRAG, which is even better than Gemini-2.0-Flash. The model shows generalization functionality beyond its training context length, and although the QWEN2-VL-72B reaches the 32K training window, the average score at 128K is 51.9.
In summary, the researchers introduced Mmlongbench, the first comprehensive benchmark for evaluating LCVLMs for various downstream tasks. It provides a rigorous basis for diagnostic cutting-edge model capabilities by covering five different task categories with uniform cross-mode token counting and standardized context length. Evaluation of 46 models shows that single-task performance inevitably predicts overall long-form cultural capabilities, while Frontier model faces enormous challenges in OCR accuracy and cross-pattern retrieval. Mmlongbench is a standard evaluation framework designed to drive future research towards more effective visual token encoding, robust position-deoxidation protocols, and improved multimodal retrieval and reasoning capabilities.
View paper and GitHub pages. All credits for this study are to the researchers on the project. Also, please stay tuned for us twitter And don’t forget to join us 95k+ ml reddit And subscribe Our newsletter.
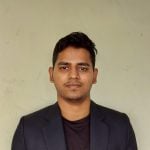
Sajjad Ansari is a final year undergraduate student from IIT Kharagpur. As a technology enthusiast, he delves into the practical application of AI, focusing on understanding AI technology and its real-world impact. He aims to express complex AI concepts in a clear and easy way.
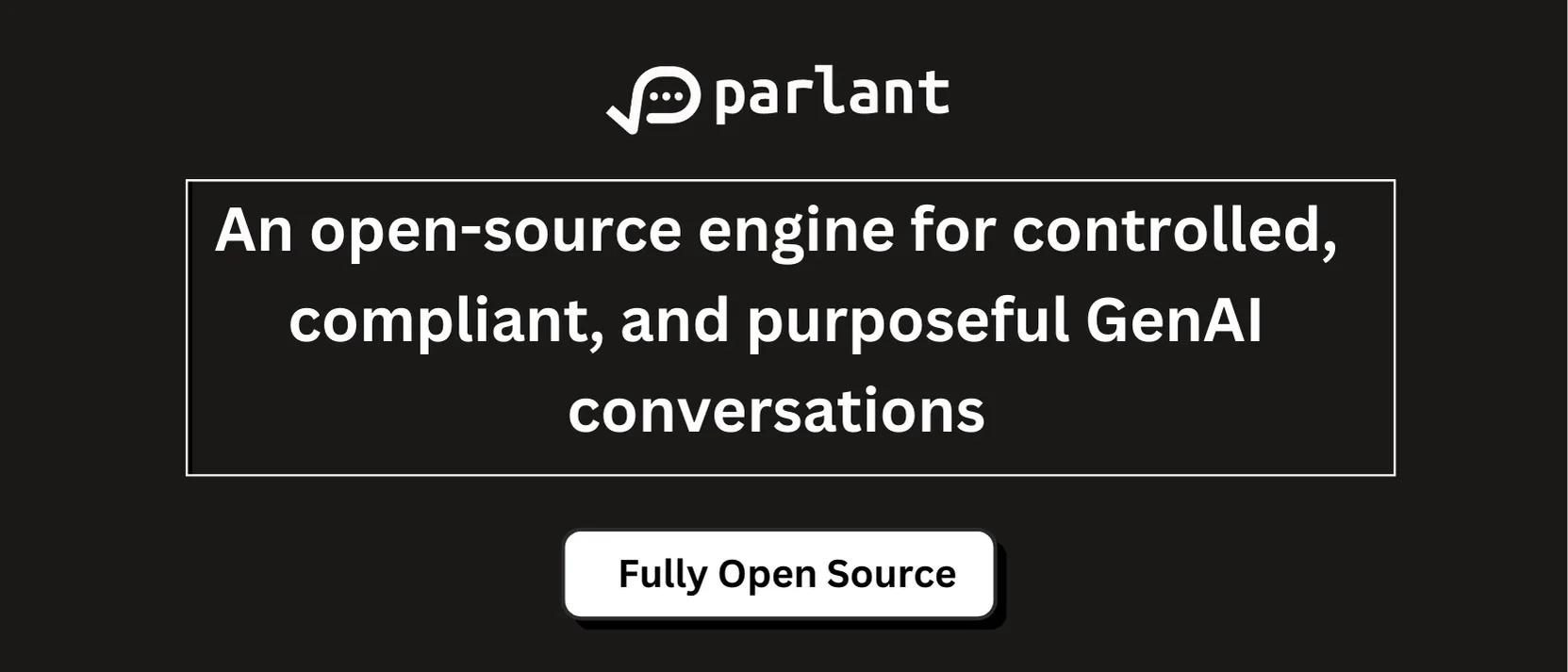