Offline video plug-in can now understand live streaming: Apple researchers introduce Streambridge to enable multi-turn and active video understanding

Video-llms handles the entire pre-recorded video at once. However, applications such as robotics and autonomous driving require causal perception and explanation of online visual information. This basic mismatch shows the limitations of current video plugins as they are not naturally designed to operate in streaming scenarios where timely understanding and responsiveness are critical. The transition from offline to streaming video understanding presents two key challenges. First, real-time understanding of multiple transformations requires models to process the latest video segments while maintaining historical visuals and dialogue environments. Second, positive response generation requires human-like behavior, the model actively monitors the visual flow and provides timely output based on the expanded content without explicit prompts.
Video-llms understands video, combining visual encoder, modal projector, and LLMS to generate contextual responses to video content. Several ways have emerged to address the challenges of streaming video comprehension. VideollMonline and Flash-Vstream introduce specialized online targets and memory architectures for processing sequential inputs. MMDUET and VISPEAK have developed special components for active response generation. Several benchmark suites have been used to evaluate streaming capabilities, including Streamingbench, Streambench, SVBENCH, OMNIMMI, and OVO BENCH.
Researchers from Apple and Fudan University have proposed Streambridge, a framework that converts offline video plug-ins to models with streaming media. It addresses two fundamental challenges of adapting existing models into online solutions: multi-transform real-time understanding and lack of proactive response mechanisms. StreamBridge combines memory buffers with circular compression strategies to support long article interactions. It also incorporates a decoupled, lightweight activation model that integrates seamlessly with existing video plugins for active response generation. Additionally, the researchers introduced Stream-it, a large dataset designed to stream video understanding, with mixed Videotext sequences and multiple illustration formats.
Evaluation was performed using mainstream offline video plugins, llava-ov-7b, qwen2-vl-7b and oryx-1.5-7b. A Stream-IT dataset of approximately 600K samples from established datasets was added to maintain general video comprehension features including Llava-178K, VCG-Plus, and ShareGPT4Video. OVO benches and flow plates are used for real-time understanding of multiple turns, with the focus on their real-time tasks. General video understanding was evaluated in seven benchmarks, including three short video datasets (MVBench, CheckTest, tempcompass) and four long-term video benchmarks (Egoschema, LongvideObench, MLVU, Videomomme).
The evaluation results show that qwen2-vl† The average score on the OVO bench increased from 55.98 to 63.35, and the average score on the flow board increased from 55.98 to 69.04. In contrast, llava-ov† The experience was slightly reduced in performance, from 64.02 to 61.64 on OVO pallets and from 71.12 to 68.39 on the flow plate. Fine-tuning on streaming datasets results in substantial improvements across all models. ORYX-1.5† Revenue of +11.92 on OVO pallet and +4.2 on flow table. In addition, qwen2-vl† After streaming, the average score on the OVO bench was 71.30 and the average score on the streaming platform was 77.04, even surpassing the performance of the GPT-4O and Gemini 1.5 Pro (such as the GPT-4O and Gemini 1.5 Pro), showing the effectiveness of Streambridge in enhancing streaming video comprehension.
In short, the researchers introduced StreamBridge, a way to convert offline video plug-ins to efficient models with streaming. Its dual innovation, memory buffer with circular compression strategies and a decoupled lightweight activation model solves the core challenges of streaming video understanding without compromising general performance. In addition, a stream data set was introduced to use a dedicated interwoven video text sequence for streaming video understanding. As streaming video understanding becomes increasingly important in robotics and autonomous driving, StreamBridge offers a popular solution that transforms static video plug-ins into dynamic, responsive systems that enable meaningful interactions in a continuously evolving visual environment.
Check Paper. All credits for this study are to the researchers on the project. Also, please feel free to follow us twitter And don’t forget to join us 90K+ ml reddit.
Here is a brief overview of what we built in Marktechpost:
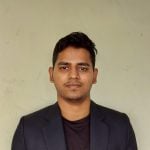
Sajjad Ansari is a final year undergraduate student from IIT Kharagpur. As a technology enthusiast, he delves into the practical application of AI, focusing on understanding AI technology and its real-world impact. He aims to express complex AI concepts in a clear and easy way.