Multimodal AI requires more than modal support: researchers propose general-level and general basis to evaluate true synergy in general models

Artificial intelligence has gone beyond language-centric systems, and it evolved into models capable of handling multiple input types, such as text, images, audio and video. The field is known as multimodal learning, aiming to replicate the natural human ability to integrate and interpret various sensory data. Unlike traditional AI models, single mode, multi-mode generalists are designed to process and cross-format responses. The goal is to create systems that mimic human cognition by seamlessly combining different types of knowledge and perceptions.
The challenge in this field is to enable these multimodal systems to prove true generalization. Although many models can process multiple inputs, they are often unable to transfer learning across tasks or ways. The lack of cross-task enhancement (called synergistic action) hinders smarter and more adaptable systems. The model can excel in image classification and text generation, respectively, but it cannot be considered a powerful generalist without the skills in both fields. Achieving this synergy is crucial to developing more capable, autonomous AI systems.
Many current tools rely heavily on the core of large language models (LLMs). These LLMs are often complemented with external dedicated components tailored for image recognition or speech analysis tasks. For example, existing models such as clips or flamingo blend language with vision, but do not connect the two in depth. Instead of acting as unified systems, they depend on loosely coupled modules that mimic multimodal intelligence. This fragmented approach means that the model lacks the internal architecture required for meaningful cross-pattern learning, resulting in isolated task performance rather than overall understanding.
Researchers at the National University of Singapore (NUS), Nanyang Technical University (NTU), Zhejiang University (ZJU), Peking University (PKU) (PKU) and other proposed AI frameworks called General Level and a benchmark called General Bench. These tools are designed to measure and facilitate synergies across models and tasks. The general level establishes five levels of classification based on the way models integrate understanding, generation and language tasks. Benchmarks are powered by General Bench, a large dataset covers over 700 tasks and 325,800 annotations examples drawn from text, images, audio, video, and 3D data.
General-level evaluation methods are based on the concept of synergy. The model is evaluated by task performance and its ability to exceed the scores of state-of-the-art (SOTA) experts. The researchers defined three types of synergy: task-to-task, understanding generation and pattern patterns, and needing to improve capabilities at each level. For example, the Level 2 model supports many ways and tasks, while the Level 4 model must show synergies between understanding and power generation. Scores are weighted to reduce bias in model advantages and encourage models to support balanced task scope.
The researchers tested 172 large models, including more than 100 best performing MLLMs, targeting general foundations. The results show that most models do not demonstrate the necessary synergy to fit advanced generalists. Even advanced models like GPT-4V and GPT-4O do not reach level 5, which requires the model to use nonverbal input to improve language understanding. The highest performing model manages only basic multimodal interactions, without one showing the overall synergy across tasks and patterns. For example, the benchmark shows 702 tasks evaluated on 145 skills, but does not achieve dominance in all areas. Using 58 evaluation metrics, General Bench’s coverage in 29 disciplines sets new standards for comprehensiveness.
This study illuminates the gap between current multimodal systems and ideal generalist models. Researchers solve the core problems in multimodal AI by introducing tools that prioritize integration over specialization. With the general level and general foundation, they provide a rigorous path forward for evaluating and building models that can handle various inputs and learn and reason in it. Their approach helps drive the domain to smarter systems with real-world flexibility and cross-pattern understanding.
Check Paper and project page. All credits for this study are to the researchers on the project. Also, please feel free to follow us twitter And don’t forget to join us 90K+ ml reddit.
Here is a brief overview of what we built in Marktechpost:
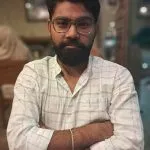
Nikhil is an intern consultant at Marktechpost. He is studying for a comprehensive material degree in integrated materials at the Haragpur Indian Technical College. Nikhil is an AI/ML enthusiast and has been studying applications in fields such as biomaterials and biomedical sciences. He has a strong background in materials science, and he is exploring new advancements and creating opportunities for contribution.